Unlocking Potential: The Role of Agriculture Dataset for Machine Learning
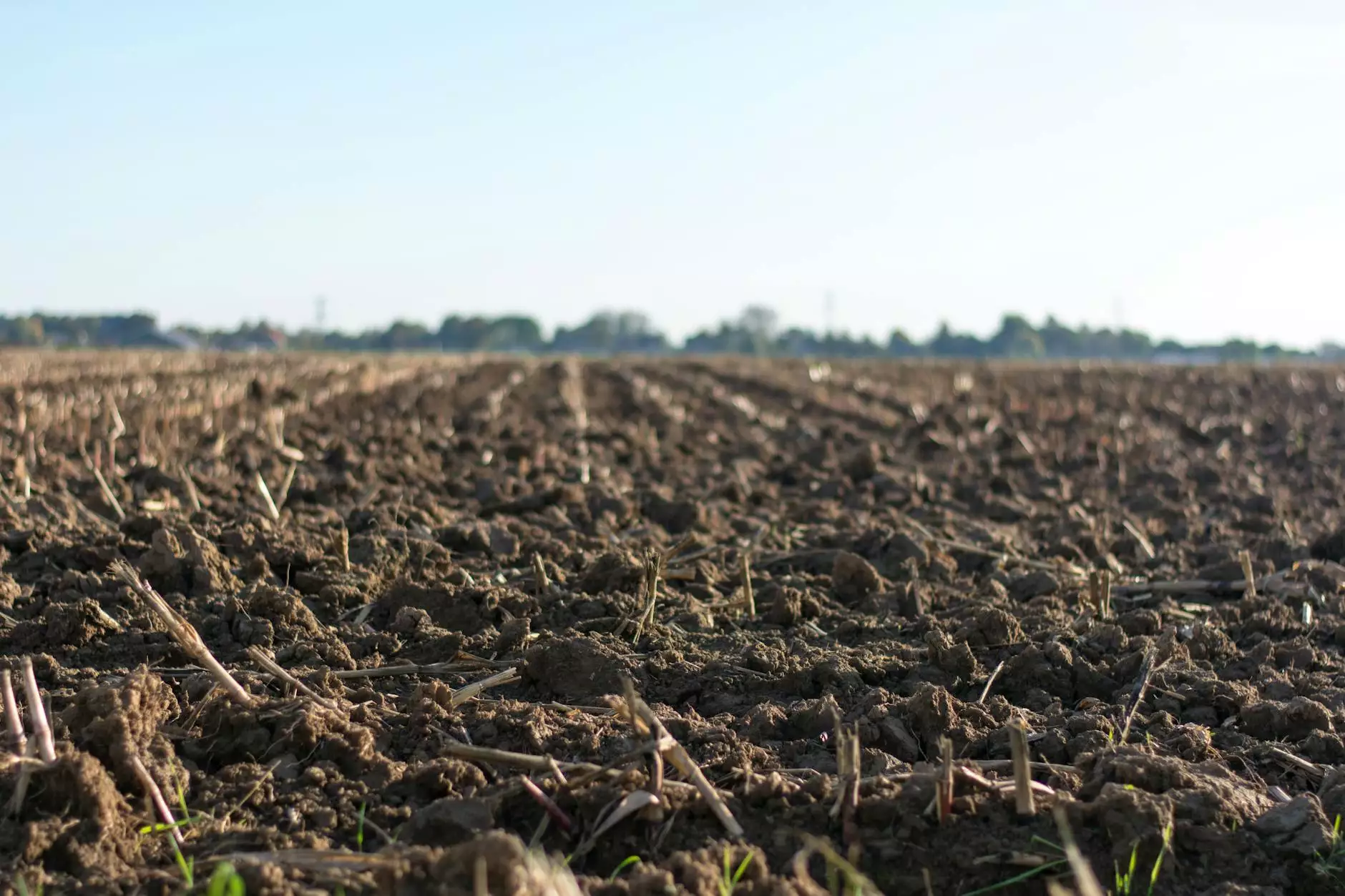
In the rapidly evolving world of agriculture, integrating technology has become a paramount necessity. The advent of machine learning within the agricultural domain brings significant advancements that can transform traditional practices into high-efficiency operations. A crucial component of this transformation is the utilization of agriculture datasets for machine learning, which act as the backbone for training models that drive innovations.
The Significance of Agriculture Datasets
Data is often regarded as the new oil in the information age, and this rings particularly true for the agricultural sector. Effective use of agriculture datasets for machine learning facilitates:
- Enhanced Decision Making: Data-driven decisions lead to better outcomes in farming operations.
- Increased Efficiency: Optimizing resource use (water, fertilizer, pesticides) improves yield while minimizing waste.
- Predictive Analysis: Farmers can predict crop yields and manage risks better through analyzed historical data.
Understanding Agriculture Datasets
Agriculture datasets can come from various sources, including:
- Remote Sensing: Satellite imagery and drones collect data on soil health, crop health, and weather patterns.
- IoT Devices: Sensors placed in fields gather real-time data regarding temperature, humidity, and soil moisture levels.
- Historical Records: Past data records help in understanding long-term trends in crop production and livestock management.
These data sources help create comprehensive datasets that machine learning models can leverage to identify patterns and predict outcomes.
Machine Learning Applications in Agriculture
Utilizing agriculture datasets for machine learning opens up a world of possibilities in rural development. Below are some notable applications:
Crop Yield Prediction
Machine learning models trained on agricultural datasets can forecast crop yields based on various factors such as weather data, soil conditions, and historical yield records. This predictive capability enables farmers to make informed decisions about planting and harvesting times.
Pest and Disease Detection
By analyzing patterns in data collected from crops, machine learning algorithms can predict pest infestations and disease outbreaks, allowing farmers to take preventive measures before significant damage occurs.
Precision Agriculture
Machine learning enables precision agriculture by analyzing data from multiple sources. This analysis allows for the precise application of resources, ensuring that farmers apply the right amount of water, fertilizers, and pesticides at the right time and place.
Supply Chain Optimization
Integrating machine learning with agriculture datasets can streamline supply chains, reducing waste and improving the efficiency of moving products from farms to consumers. This includes optimizing routes, predicting demand, and managing inventory levels effectively.
Challenges of Using Agriculture Datasets
While the use of agriculture datasets for machine learning holds immense potential, it also comes with challenges:
- Data Quality: Ensuring that the data collected is accurate, timely, and relevant is crucial for producing reliable models.
- Integration of Diverse Data Sources: Combining data from various platforms and formats can be complex and requires robust data management strategies.
- Skill Gap: There is often a lack of trained personnel who can analyze and interpret complex data insights effectively.
The Future of Agriculture Through Data and Machine Learning
The future of agriculture lies at the intersection of technology and tradition. With the continuous development of machine learning algorithms and the ever-increasing amount of available data, the potential for innovation is limitless. Farmers who embrace these technologies stand to benefit significantly in the following ways:
Sustainability in Farming Practices
By utilizing data analytics, farmers can adopt more sustainable practices that preserve natural resources. Machine learning allows for reducing overuse of chemicals, optimizing irrigation practices, and improving energy efficiency.
Global Food Security
As the global population continues to rise, food security becomes increasingly important. Machine learning applications can help increase yields and reduce losses, contributing to food availability and affordability.
Improved Economic Viability
Farmers using data-driven techniques can realize significant cost savings and improved margins through efficient practices. This enhances the overall economic viability of agricultural operations.
Case Studies: Success Stories in Agriculture
Across the globe, various farms and agricultural businesses have successfully implemented agriculture datasets for machine learning, leading to remarkable outcomes. Here are a few notable examples:
1. The Case of John Deere
John Deere has embraced machine learning by integrating advanced data analytics in its Precision Agriculture initiative. By utilizing real-time data collection through its machinery, the company enables farmers to make informed decisions that significantly enhance crop yield and resource management.
2. IBM Watson’s Agricultural Innovations
IBM’s Watson has been pivotal in providing machine learning solutions to farmers to analyze weather patterns, soil conditions, and crop health. This integration has allowed for timely interventions that optimize growth and minimize losses.
3. The Growers Network's Use Case
Growers Network has leveraged machine learning to create a framework for farmers to share data and insights. This community-driven approach allows farmers to optimize their practices collectively, leading to better harvests and reduced costs.
Conclusion: The Road Ahead
The journey towards integrating agriculture datasets for machine learning is an exciting one. As innovations continue to unfold, the agricultural sector stands on the brink of transformation that promises higher efficiency, sustainability, and profitability. With the barriers of data quality and integration being addressed, a future where technology and agriculture work hand in hand is not just a possibility; it is becoming a reality.
Farms that adopt these advanced technologies will not only enhance their productivity but also contribute toward building a sustainable future for global agriculture. As we move forward, it is imperative that all stakeholders in the agriculture industry— from farmers to policymakers—recognize the value of data and invest in the necessary technologies to harness its full potential.
agriculture dataset for machine learning