Machine Learning in Malware Detection: The Future of Cybersecurity
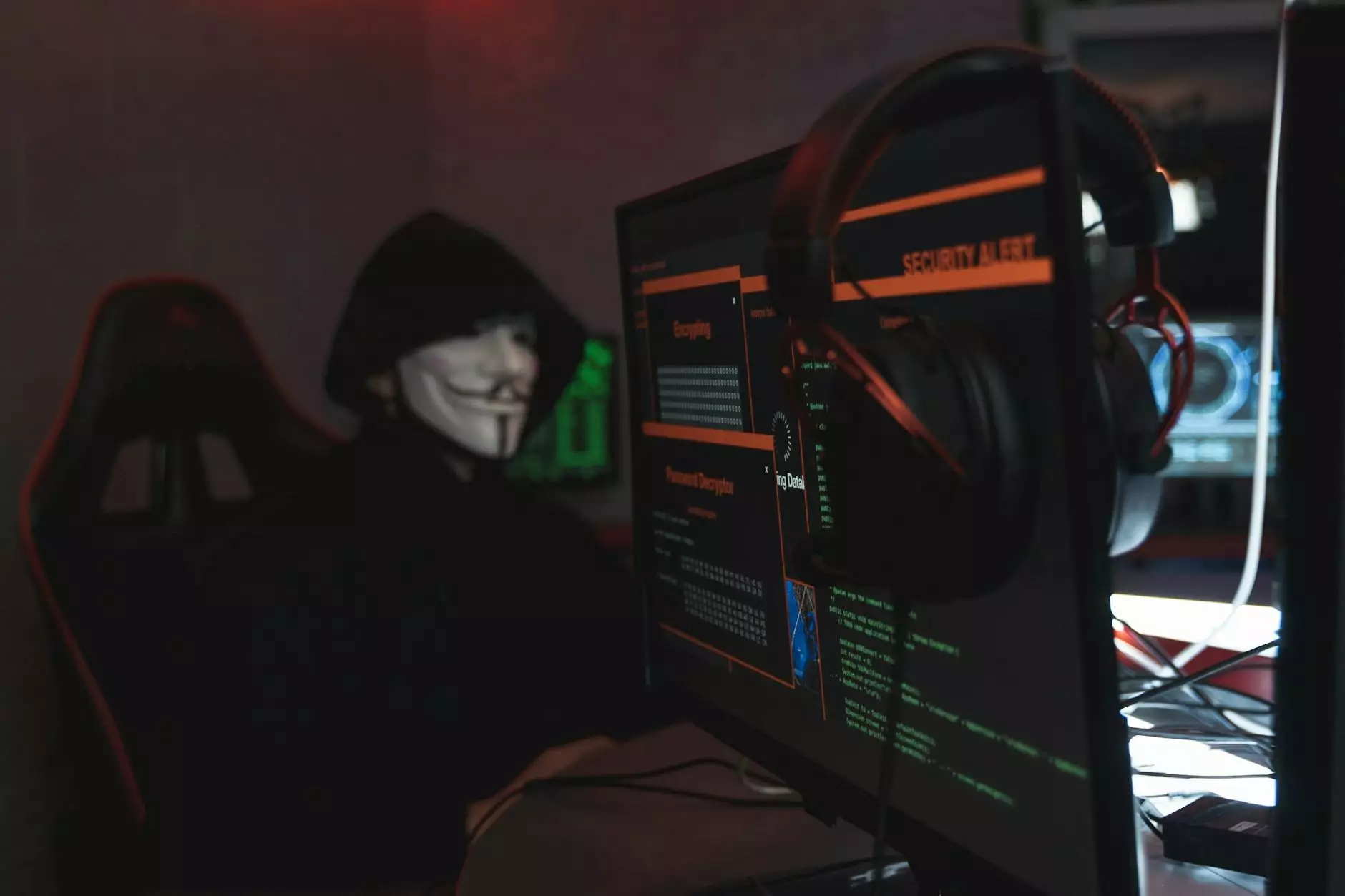
Understanding Malware and Its Implications
Malware, short for malicious software, encompasses a range of hostile software from viruses and worms to more complex forms such as ransomware and spyware. The increasing sophistication of these threats poses significant challenges for businesses, particularly in their IT services and computer repair sectors. Understanding how malware operates and the threats it poses is crucial for developing effective detection and response strategies.
As technology advances, so does the ingenuity of cybercriminals. The global cybersecurity landscape is in a constant state of evolution, which necessitates the implementation of innovative solutions like machine learning to mitigate risks associated with malware.
The Role of Machine Learning in Cybersecurity
Machine learning (ML) has emerged as a transformative technology in the realm of cybersecurity. By leveraging algorithms and statistical models, machine learning systems can learn from data and improve their predictive capabilities without explicit programming. This adaptive learning is particularly beneficial in detecting emerging malware threats.
How Machine Learning Works in Malware Detection
Machine learning algorithms analyze vast amounts of data to identify patterns and anomalies that indicate the presence of malware. Here are some key processes involved:
- Data Collection: Gathering data from numerous sources, including network traffic, user behavior, and system events.
- Feature Extraction: Identifying relevant features from the data that can distinguish between normal and malicious activities.
- Model Training: Using labeled datasets (examples of benign and malicious behavior) to train the machine learning models.
- Prediction: Once trained, these models can predict new threats based on learned patterns.
- Continuous Learning: The ability to update models as new data becomes available ensures that the system adapts to new threats.
Benefits of Implementing Machine Learning in Malware Detection
The integration of machine learning into malware detection provides several compelling advantages:
1. Enhanced Detection Rates
Machine learning algorithms can analyze and compare vast datasets at incredible speeds, significantly improving detection rates of sophisticated malware that traditional methods may miss.
2. Reduced False Positives
High false positive rates can overwhelm security teams. Machine learning helps to refine detection accuracy, focusing on genuine threats and minimizing false alarms.
3. Real-Time Threat Analysis
Machine learning systems can analyze incoming data in real-time, allowing for immediate response to suspected malware activities. This is critical for mitigating potential damages before they escalate.
4. Predictive Capabilities
Utilizing historical data, machine learning algorithms can forecast potential attack vectors, empowering organizations to proactively bolster defenses against emerging threats.
Challenges in Implementing Machine Learning for Malware Detection
Despite the numerous benefits, there are challenges that organizations must navigate when implementing machine learning solutions for malware detection:
1. Data Privacy and Compliance
As businesses collect extensive data for machine learning, they must ensure compliance with regulations like GDPR, which can complicate data management practices.
2. Model Interpretability
Many machine learning models act as "black boxes," making it difficult for cybersecurity professionals to understand the reasoning behind specific alerts. This lack of transparency can hinder effective incident response.
3. Resource Intensive
Building and maintaining machine learning systems require significant computational resources and expertise, which may be a barrier for smaller organizations.
4. Evolving Malware Strategies
As malware authors adapt to new defense mechanisms, machine learning models must also evolve, necessitating continuous updates and retraining to stay effective.
Strategies for Effective Machine Learning in Malware Detection
To successfully implement machine learning in malware detection, organizations should consider the following strategies:
1. Establish a Robust Data Collection Framework
Implement comprehensive data collection mechanisms to gather relevant historical and real-time data, ensuring diverse datasets that enhance model training.
2. Collaborate with Cybersecurity Experts
Incorporating insights from cybersecurity professionals can help in developing models that align closely with actual threat landscapes, improving detection accuracy.
3. Invest in Continuous Training and Testing
Regularly retrain machine learning models with fresh datasets to account for emerging malware threats and evolving attack strategies.
4. Enhance Model Transparency
Utilizing explainable AI techniques can provide insights into the decision-making processes of machine learning models, fostering trust and understanding in their outputs.
Conclusion: Embracing the Future of Cybersecurity
The integration of machine learning in malware detection represents a pioneering leap in cybersecurity, enabling organizations to combat malware threats more efficiently and effectively. As the landscape of cyber threats continues to evolve, the marriage of advanced technologies like machine learning with practical security strategies will be essential in safeguarding digital assets. Companies like Spambrella are at the forefront, championing innovative solutions to enhance security systems and IT services. By prioritizing the implementation of machine learning capabilities, organizations can not only protect their operations today but also prepare for the challenges of tomorrow.
For more information and expert assistance in strengthening your cybersecurity measures, visit Spambrella.com.